Social Media Information Can Predict a Wide Range of Personality Traits and Attributes
– Findings could bring new technologies to mental health diagnostics and personalized nudges –
August 24, 2020
National Institute of Information and Communications Technology
Highlights
- Wide personality traits and attributes such as extraversion and IQ were predicted from Twitter information
- Network and linguistic information from Twitter usage predicts social personality and mental health
- The findings could lead to new technologies for mental health diagnostics and personalized nudges
Abstract
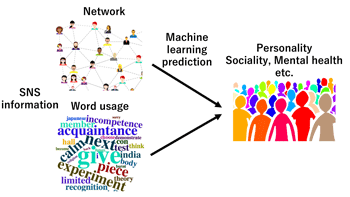
Background
Achievements
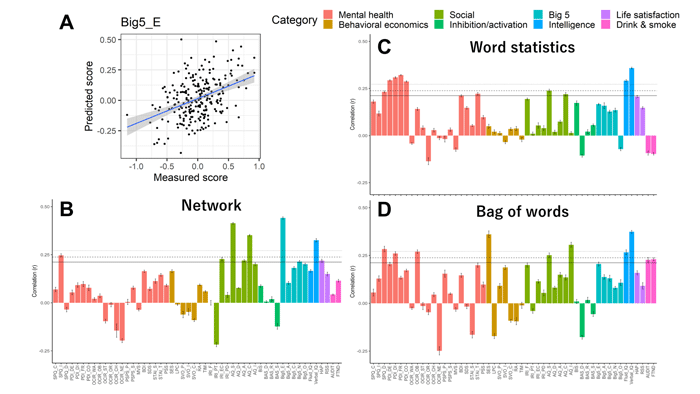
A: measured and predicted scores of Big5 extraversion. B: predictions from network infotmation.
C: prediction from word statistics. D: prediction from word usage (bag of words).
Performance was evaluated by correlation coefficinets between measured personality and attribute scores and predicted ones. Solid, dashed and dotted lines show p = 0.05/52, p = 0.01/52, and p = 0.001/52, respectively.
[Click picture to enlarge]
Future Prospects
Paper details
Funders
Appendix
Details of the experimental findings
Personalities traits and attributes
Predictions from network features
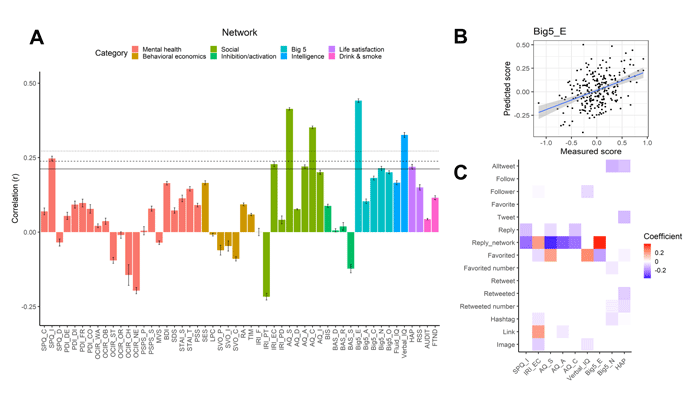
[Click picture to enlarge]
Predictions from time
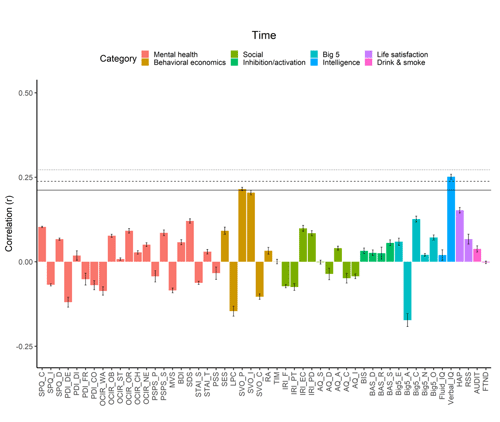
[Click picture to enlarge]
Predictions from word statistics
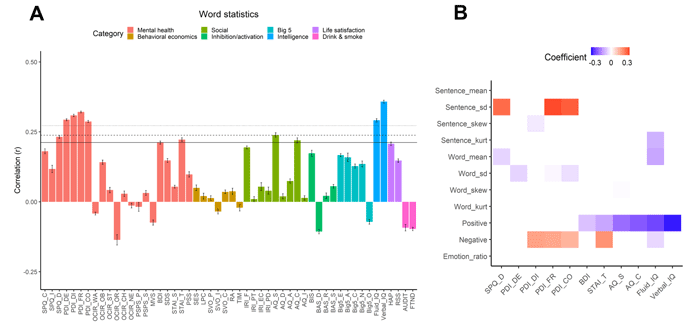
[Click picture to enlarge]
Predictions from word usage
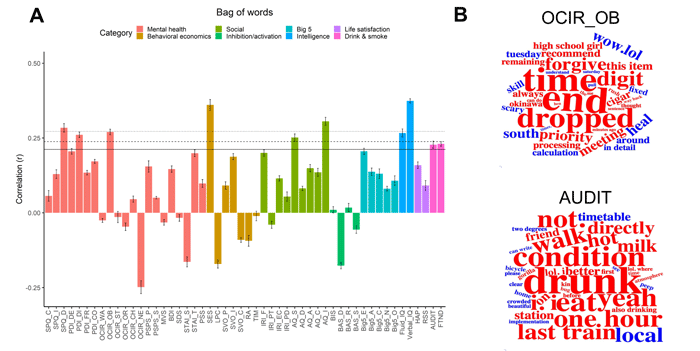
[Click picture to enlarge]
Conclusion
Glossary
Technical Contact
HARUNO Masahiko
Neural Information Engineering Laboratory
Center for Information and Neural Networks
NICT
Tel: +81-80-9098-3239
E-mail:
Media Contact
HIROTA Sachiko
Press Office
Public Relations Department
NICT
E-mail: